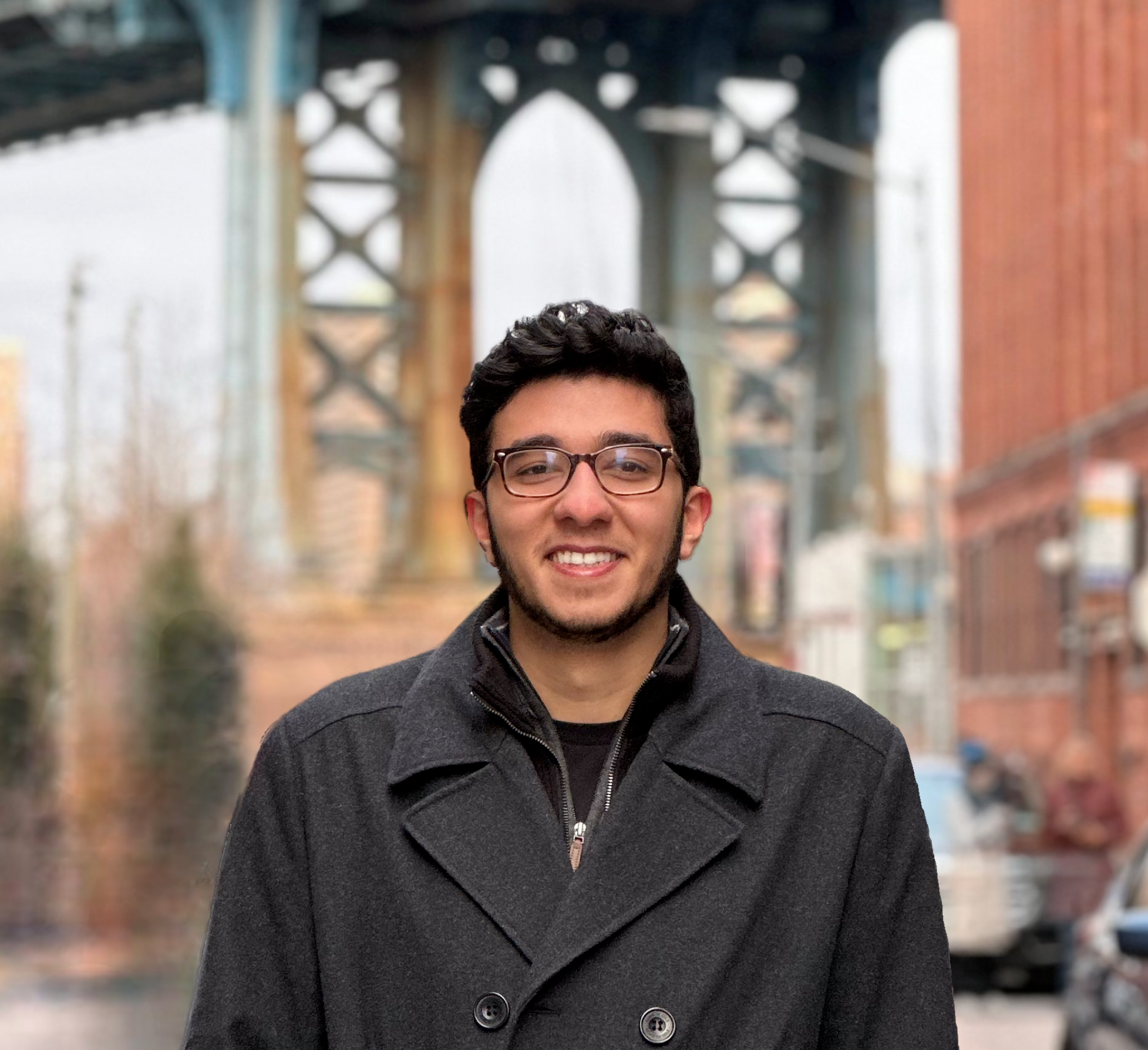
I'm Ahmad Abdel-Azim.
Studying statistics and molecular & cellular biology at Harvard
I develop and implement novel statistical methods to overcome challenges in genomic medicine, all while collaborating in fast-paced, impact-oriented, and innovative research environments.
I am driven to apply computational and statistical techniques to advance the prospect of precision medicine and design patient-tailored therapies for the treatment of human diseases. Toward that goal, I have worked as a researcher and intern at several academic labs, companies, and pharmaceutical startups. At Harvard, I am currently pursuing an A.B./A.M. concurrent degree in statistics and molecular & cellular biology.
This website is a collection of my projects and professional experiences. I also write about various topics in statistics and deep learning here!
Experience
Current |
![]() |
HSPH | Researcher, Xihong Lin Lab @ Harvard | 2022 - Present | hsph.harvard.edu/lin-lab/ |
Former
![]() |
Regeneron | Intern, Regeneron Genetics Center | 2023 - 2023 | regeneron.com | |||
![]() |
Biotia | Bioinformatics Consultant | 2022 - 2023 | biotia.io | |||
![]() |
BWH | Researcher, Kwiatkowski Lab @ Harvard | 2020 - 2022 | kwiatkowskilab.org | |||
![]() |
MIT CSAIL | Research Student, Manolis Kellis Lab @ MIT (6.047) | 2021 - 2021 | web.mit.edu/manoli | |||
![]() |
HMS | Researcher, Debora Marks Lab @ Harvard | 2019 - 2020 | deboramarkslab.com |
Teaching
![]() |
STAT 117 | Teaching Fellow, Statistics Dept. (Harvard) | Spring 2024 | harvard.edu/statistics-courses | |||
![]() |
STAT 110 | Teaching Fellow, Statistics Dept. (Harvard) | Fall 2023 | harvard.edu/stat110 | |||
![]() |
STAT 185 | Teaching Fellow, Statistics Dept. (Harvard) | Fall 2023 | harvard.edu/statistics-courses | |||
![]() |
STAT 111 | Teaching Fellow, Statistics Dept. (Harvard) | Spring 2023 | harvard.edu/statistics-courses | |||
![]() |
MCB 112 | Course Assistant, MCB Dept. (Harvard) | Fall 2022 | mcb112.org |
Selected Projects
Developing polygenic risk scores for correlated data structures.
We introduce a unified statistical framework for polygenic risk score prediction in the presence of correlated data, leveraging mixed effects modeling. We are evaluating the performance of this new approach via simulation across several genetic architectures. We further apply our approach to compute PRS for common complex diseases in the UK Biobank and demonstrate the potential of our method for clinical translation of polygenic prediction.
Links: GitHub, Project Page
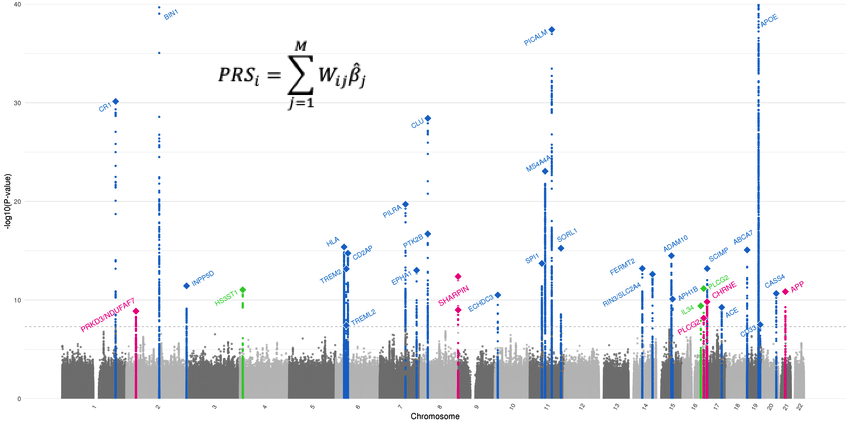
Integrating multiple data modalities for accurate diagnosis.
Applying deep learning techniques to to predict Alzheimer's disease (AD) onset and progression from genetic, neuroimaging, and clinical data to facilitate rapid and precise diagnosis. We employ XGBoost models to select important genomic features, build 3D convolutional neural network (CNN) for MRI data, and design a classifier to combine retained features from both models with clinical information. With a focus on accuracy and predictive capabilities, the method shows promise for early detection.
Links: GitHub, Project Page
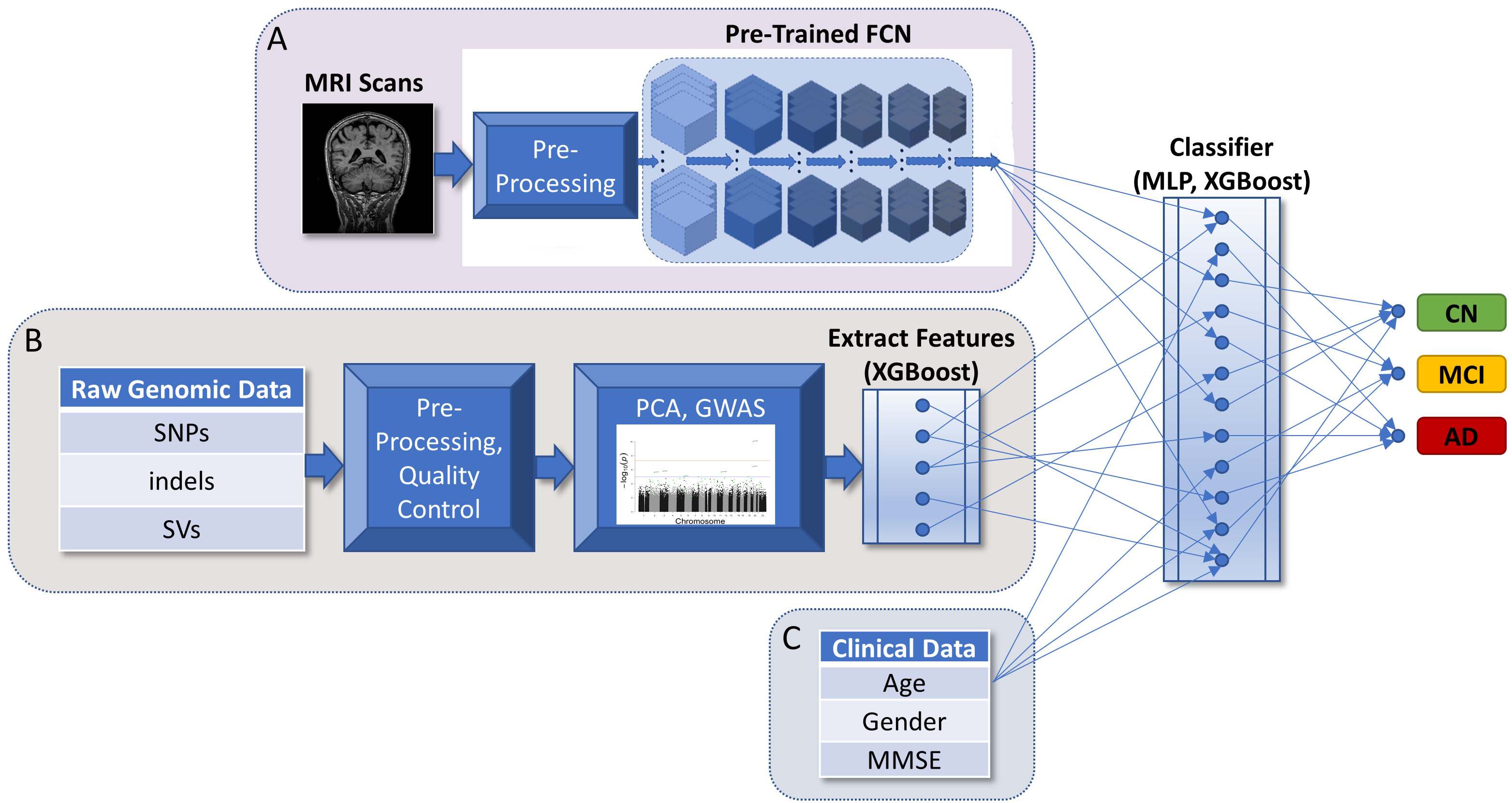
Building a Gibbs sampler to aggregate expert insights.
We present a comprehensive statistical framework for analyzing expert rankings of NFL quarterbacks. The approach utilizes latent regression models, specifically designed to handle the idiosyncrasies and biases inherent in expert evaluations. We derive and implement a Gibbs sampler in R, infering a consensus ranking from multiple experts, while accounting for missing data and heterogeneity in the expert opinions. The statistical method prioritizes the exploration of underlying patterns in the data over the application of rankings, offering a robust tool for similar analyses in other domains.
Links: GitHub, Project Page
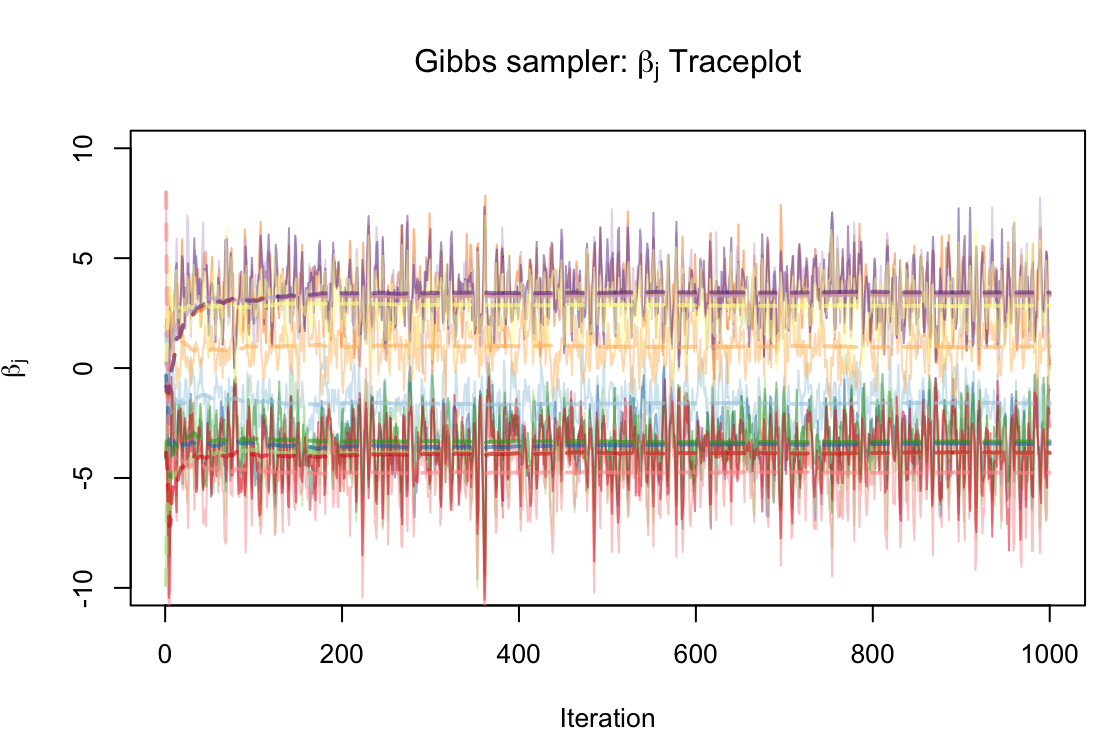